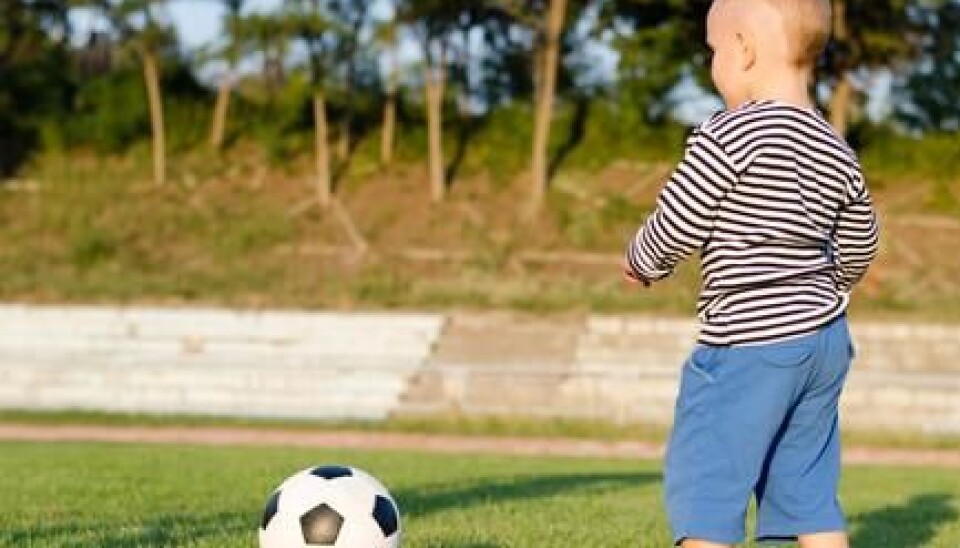
How childplay inspired new artificial brain
We can throw and catch a ball because our brain uses patterns to recognise situations and react to them. This form of pattern recognition is now being used to develop an artificial brain, which in record time can simulate buildings and produce a fatigue life estimation.
When we humans move around, our brain is working flat out to calculate risks, predict other people’s movements and decide the best overall strategy for reaching the desired goal.
Many of these calculations occur so quickly that we are not conscious of them.
When children play dodgeball in the school yard, they throw, catch and dodge balls with great ease. Calculating the ball’s trajectory through the air would, however, require a set of highly complex equations.
If the human brain were to perform these calculations the same way as a computer would, there would be no catching or throwing of balls in the school yard. We would simply take too long to calculate and predict the movement of the ball to react in time.
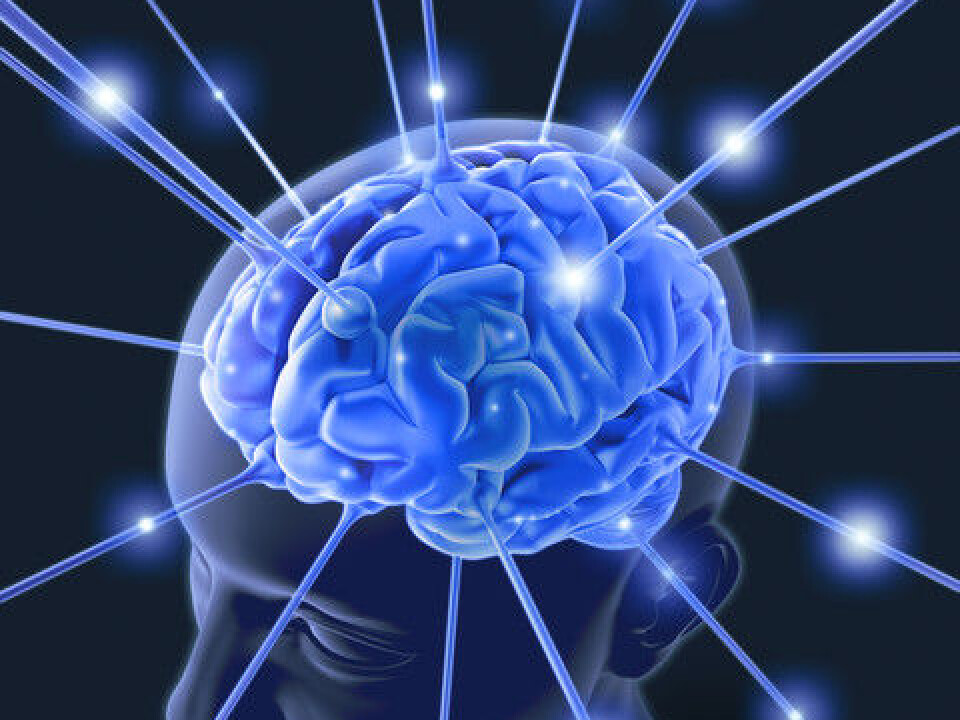
Fortunately, the human brain does not calculate with equations. It uses patterns instead. And even though individual processes are processed much faster by a computer than by a human brain, the brain is vastly superior when it comes to pattern recognition.
The brain predicts patterns at incredible speeds
The human brain consists of billions of neurons that are connected through a great number of synapses. This structure enables us to process and react to sensory impressions with stunning speed.
Once the brain has learned a pattern, it can recognise and predict this pattern with great speed. It is this ability that enables us to predict the movement of a ball, so that we, in a fraction of a second, can decide between catching or dodging the ball.
This ability also makes it possible for us to recognise faces and read handwriting. The ability can be replicated mathematically. The method is called ‘neural networks’ and is one of the tools we generally refer to as artificial intelligence.
Neural networks identify patterns in large datasets
Neural networks represent what in popular terms is known as the unconscious part of the brain.
Most of the patterns we see are processed so quickly that the process occurs unconsciously, meaning that we often don’t get the chance to suppress it. It is, for instance, impossible to say in advance whether or not you will be able to recognise an old acquaintance if you were to bump into him or her on the street.
From an engineering point of view, the great advantage of these neural networks is that they can identify patterns in large datasets. Not only that; once the pattern has been identified, the networks can also make predictions at the same impressive speed as the human brain.
Neural networks in structural design
A Danish research project has now set out to develop a method in which neural networks are used to simulate the movements of building structures.
The movements of a building structure over long periods are used to predict the fatigue life of the structure. The idea is to combine traditional simulation techniques with the use of neural networks.
The traditional methods currently being used often require highly sophisticated calculation models. These models make heavy demands on the computational power and thus also on the computing capacity.
Small, frequent loads are hard to calculate
Strength calculations in structural design can be divided into two disciplines: extreme situations and fatigue.
Extreme situations are intense but rare events such as hurricanes, torrential rain, earthquakes, explosions and collisions.
Fatigue is small, frequent loads such as wind, waves, traffic and pedestrians, which wear on the structures over time.
The fatigue analyses are particularly difficult to compute and thus also time-consuming.
However, since structures often move in a fixed pattern, neural networks can be trained to recognise this pattern and can then be used to shorten the calculation time.
Network is trained like a child
In short, the new method consists of first setting up a detailed calculation model of the structure in the traditional way.
This model is used for performing a short simulation. It is these simulations that are time-consuming, so the simulation needs to be as short as possible, yet long enough to determine the dynamic properties of the structure, i.e. the small movements that it is exposed to on a daily basis.
The data that comes out of this simulation contains the structure’s movement patterns. By using this data to train the neural network, we end up with a small artificial brain, which in the blink of an eye can predict how the structure will respond to a given external load.
This training of the neural network, with data that has been calculated in advance, corresponds to letting a child see many balls flying through the air one by one. After relatively few balls, the child will be able to catch the balls because it can recognise the movement pattern of the balls.
New method bypasses annoying dilemma
A good design foundation for structures basically requires two things – a detailed and refined calculation model and an extensive analysis. This presents us with a dilemma: the better and the more refined the calculation models get, the greater the demands on computing power for the subsequent analyses.
This means that one must always look for a compromise between the degree of detail and the extent of the analysis, because when the calculation models become highly detailed, the analyses become so computationally intensive that even with the greatest computing power, the analyses would be impossible to carry out in practice.
The new method has a way of bypassing this dilemma, as the neural network can learn the dynamic properties of the structure and at the same time perform high-speed calculations.
Huge savings in computation time
The method has been tested on an anchoring system on a large sea installation.
With this method it is possible to perform simulations that would normally take up to ten hours to compute in less than two minutes.
This is a fundamentally different way of calculating – a method which provides a revolutionary reduction in computing time.
It also serves as a reminder of what a fantastic instrument the human brain actually is.
--------------------------
Read the Danish version of this article at videnskab.dk
Translated by: Dann Vinther