Researchers' Zone:
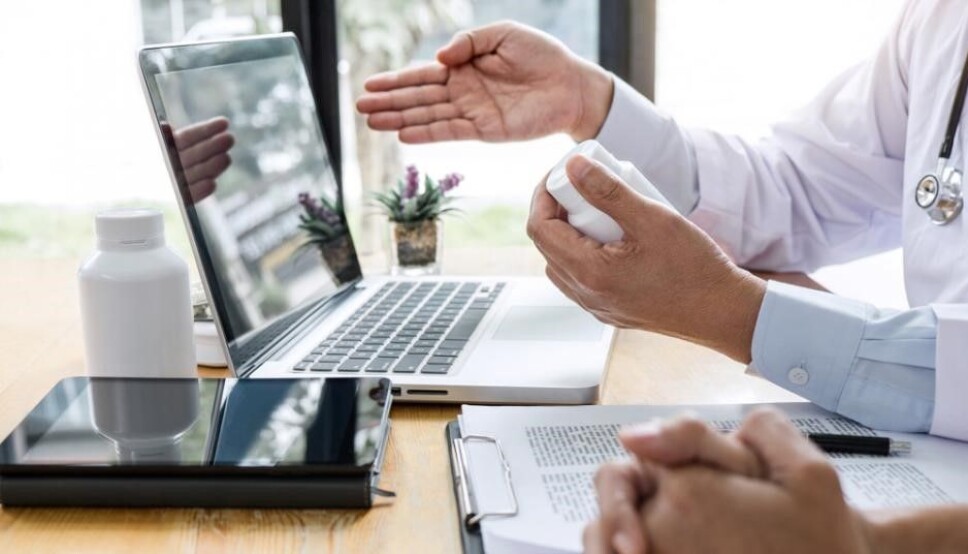
Science is more than applied statistics – can we be freed from p-value tyranny, please!
COMMENT: It is nice when complex things can be reduced to something simpler. But sometimes you lose more than you gain – such as, for example, when scientific studies are violently shoehorned into two simple boxes: ‘significant’ and ‘nonsignificant’.
How likely is it that a scientific finding – for example, that exercise has a positive effect on blood pressure – is actually just a statistical coincidence?
How sure can we be that a given treatment actually works?
Across much of science, the p-value is used to answer that question. If it is sufficiently low, the scientific finding is considered to be 'significant' – that is, not a coincidence.
If, on the other hand, it is too high, the results are written off as nonsignificant and therefore not to be trusted.
However, this distinction between useful and useless findings is far from unproblematic.
In a recent commentary published in the journal Nature, the authors and more than 800 co-signatories call for a revolt against the widespread practice of drawing conclusions on a research project based primarily on the p-values it obtained.
They argue that the narrow focus on p-values too often leads to the rejection of important findings as being nonsignificant.
They also point out that the very same findings are described differently depending on whether they were 'significant' or not.
In short: they believe that p-values do more harm than good – and we agree!
What is a p-value?
Interpreting the results of scientific trials is not always straightforward.
As a researcher, there is a challenge in determining whether a given research result reflects a real effect or is the result of chance and other factors that have nothing to do with the tested treatment.
This is where the so-called p-value and the concept of statistical significance play a role. This is not the place for a detailed account of the mathematics behind it, but the p-value expresses the likelihood that one would have found what one has now found (for example, a treatment’s effect) if (and this is a very important 'if') we assume that there is in fact no effect.
The lower the p-value, the lower the probability that the demonstrated effect is due to chance.
Here it is important to tread carefully.
For the p-value should not be confused with the likelihood that something has been found that is not really correct (a so-called false positive finding). Confused? Most are.
In fact, many researchers would probably wince if they were asked to explain what a p-value is.
The trick lies in p-value’s built-in assumption that there is actually no correlation (e.g., a treatment’s effect); an assumption that does not necessarily hold.
So, one more time for luck; or, as we say in Denmark, ‘one more time for Prince Canute’:
The p-value is 'the probability that the effect you are witnessing is a coincidence if we assume there is no real effect'. And that is not the same as 'the probability that the effect you have found is wrong.'
Or with an example: If you find that drug A works better than drug B, the p-value gives you the probability that your finding is a coincidence if we assume that drugs A and B in reality work equally well.
The concept of statistical significance is closely linked to the p-value.
It is said that a result is statistically significant, i.e., not a coincidence, when the p-value is lower than a predefined limit.
In medical research, this is typically set at 0.05.
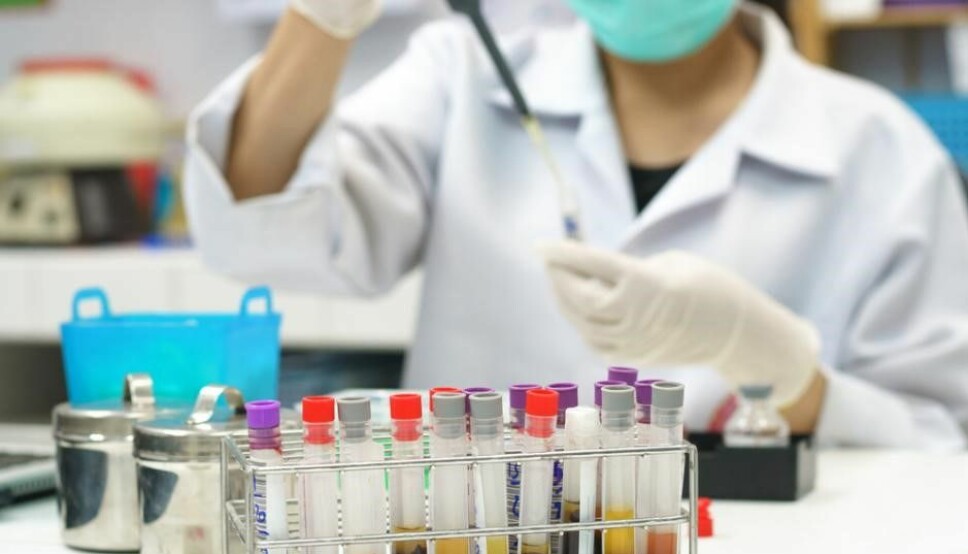
Change on the way after years of debate?
There is growing momentum behind the move away from an interpretive practice based on p-values.
This discussion is far from new. For example, the problems with p-values are brilliantly described in this nearly 20-year-old educational article from The BMJ.
However, the debate has really taken off in recent years.
This is mainly due to the much-discussed statement from the American Statistical Association in 2016 about the use and abuse of p-values, in which they explain in very clear terms what a p-value can and cannot be used for.
The debate has flared up again after The American Statistician journal published a special issue with 43 articles under the title, ’Statistical Inference in the 21st Century: A World Beyond p<0.05.’
It was the publication of this special issue that led to the commentary in Nature.
It seems, therefore, that change may actually be on the way.
So, what is so wrong with that p-value?
The fundamental problem is that the p-value does not really give us the information we are interested in.
We want to know the probability that a given research result is a true/false positive. And you cannot read that from the p-value.
Completely defining significance on the basis of p-values comes with the risk of mistakenly accepting that there is a real effect (a so-called type 1 error) or mistakenly rejecting that there is a real effect (a so-called type 2 error).
But what do these type 1 and type 2 errors look like in practice?
Type 2 error: when the p-value is used to reject something important...
A narrow interpretation of p-values can lead to the rejection of an effect if the p-value is simply greater than the notorious 0.05.
Unfortunately, this often happens despite the fact that the observed effect clearly indicates that the newly tested treatment actually provides a meaningful benefit.
Let us take an example: in a recent (and very large and thorough!) study published in the journal JAMA, a test was undertaken as to whether aggressive blood pressure treatment offers protection against dementia.
At the end of the study, aggressive blood pressure treatment was found to reduce the incidence of dementia by 17 percent compared to regular blood pressure treatment.
However, this difference is not statistically significant as the p value, by a whisker, does not fall below 0.05.
Therefore, the authors conclude that ‘intensive blood pressure control did not significantly reduce the risk of probable dementia’.
Note here how 'statistical significance' is referred to simply as 'significance'.
Most will therefore read this study as if the extra blood pressure treatment does not impact the risk of dementia to a ‘significant degree’. But this is clearly nonsense, given that the most likely scenario is that there is a 17 per cent reduction.
P-value tyranny of the worst kind
To make matters worse, the same study concludes that an effect is observed on the incidence of ‘the combined rate of mild cognitive impairment or probable dementia’.
Although this is a slightly smaller effect with a 15 percent reduction in incidence, the p-value here just creeps below 0.05, so the conclusion is qualitatively quite different.
Thus, despite exciting and quite convincing findings, we have suddenly talked ourselves out of the fact that the treatment shows beneficial outcomes.
This is p-value tyranny of the worst kind. And, unfortunately, this is not an isolated occurrence.
For example, a recent study in the New England Journal of Medicine concluded that, ‘Antibiotic prophylaxis before miscarriage surgery did not result in a significantly lower risk of pelvic infection’, as the p-value was 0.09 – despite a 23 percent relative reduction in the incidence of infections.
And an earlier study in JAMA concluded that an impressive 30 percent reduced incidence of cancer was not 'a significantly lower risk' as the p-value was 0.06.
Does the p-value have a justification?
It is quite reasonable to argue that it is important to be critical of new findings.
If the above examples had been industry-sponsored studies of new drugs, then a predefined cut-off, such as the 0.05, would probably be more greatly appreciated in order to prevent insufficiently substantiated claims of an effect.
Similarly, conservative interpretation can block coincidental findings, thus saving resources from being thrown at unfruitful research questions.
Against this background, some researchers have argued recently for a decidedly strict p-value concept with a cut-off of 0.005.
The same researchers are also highly critical of the Nature commentary.
We certainly also believe that it is important to be conservative in assessing new findings.
However, this is not in itself an argument for using the p-value as a cut-off.
Instead, efforts should be made to set targets for clinically meaningful output ratings and a more graduated understanding of evidence.
However, before we embark on alternatives to p-values, we must, paradoxically, first deal with another and diametrically opposed problem, namely the misuse of the p-value to support correlation claims.
Type 1 error: when the p-value is used to maintain nonsense...
Unfortunately, just as the p-value can be used to reject otherwise meaningful correlations, it is also often the case that it can be used as an argument for supporting claims that appear unfounded when considered in light of the overall knowledge.
This, too, is most easily illustrated by an example:
In 2014, a small study reported an apparent link between Viagra use and the risk of developing melanoma.
Although the study involved very few cases of melanoma, it just managed to obtain statistical significance and was published in the prestigious journal JAMA Internal Medicine.
The hypothesis that Viagra use could cause melanoma is actually not as far-fetched as one would think, since sildenafil (the active ingredient in Viagra) actually affects a signalling pathway in the cells involved in how melanoma can spread.
Several research groups therefore decided to replicate the finding of an increased risk.
This led to four quite solid studies from Sweden, the UK, another one from the UK (based on the same data) and Denmark.
The four groups reached broadly identical conclusions: there seems to be a slight increase in risk, but this does not represent a true correlation.
Rather, the explanation is that Viagra users (compared to non-users) go to the doctor more often (and thus have a greater chance of having their melanoma detected) and probably also have slightly different sunbathing habits.
This all sounds good, so surely that hypothesis has been put to bed? Unfortunately not...
Distorted evidence
Shortly after the four studies came out, a so-called meta-analysis was published that summarised the findings from the four articles.
To the massive frustration of the authors who wrote the four studies discussed above, the authors of the meta-analysis conclude that, across the four studies, an increased relative risk of melanoma was approximately 12 percent (between 3 and 21 percent).
And the authors of the meta-analysis conclude that this supports the understanding that there is a link – alongside the obligatory 'more studies should therefore be carried out'.
Here, contrary to the conclusions of the four studies that the analysis is based on, it is concluded that sildenafil and similar substances lead to melanoma.
The primary argument is not so much the 12 percent (which, incidentally, is a very small risk increase) but rather that the lower limit of the estimate is three percent, and since it is a shade above 0, the p-value comes in at just under 0.05.
This is but one example that clearly demonstrates how a p-value-based interpretative framework ends up with all relevant elements of a phenomenon being ignored, thereby leading to a conclusion that is contrary to reality.
This is not only a massive waste of research resources; when future advice is based on a distorted representation of the evidence, it is also detrimental to patients.
The yes/no mindset is too simple
Complex problems can rarely be solved with simple measures.
It is, therefore, not entirely easy to answer what we should use instead to test whether something is 'statistically significant'.
The fundamental problem of distinguishing real effects from 'statistical noise' will always exist.
This is why one cannot get around statistical analyses in the form of p-values, confidence intervals and power, for example.
Nevertheless, the simple 'yes/no' approach that lies in the concept of 'statistical significance' is meaningless and should therefore be avoided.
Evidence must be based on holistic considerations
However, we still have to take into account the plausibility of studies.
If you know the plausibility – that is, the likelihood that a treatment will work – you can calculate the probability that a given result is a false positive, i.e., the probability that a given result is not true.
The problem with this, of course, is that plausibility in almost all cases must be based on an assessment.
One possibility could be to report the probability of a false positive result if the plausibility is at 50 percent, for example.
This is, of course, also an arbitrary choice. Nevertheless, it would be more informative than an assertion of a statistically significant/non-significant result based on a p-value.
Whatever prevails in the future, it is important to insist on assessing the evidence holistically.
In other words, taking into account methodology, plausibility, etc. Because science is, to say it once again, more than just applied statistics.
Read this article in Danish at Videnskab.dk's Forskerzonen.
References
Anton Pottegård’s profile (SDU)
Jan Lindebjerg’s profile (SDU)
Commentary: Scientists rise up against statistical significance. (Nature)